Gartner estimates that by 2025, over 50% of large enterprises will deploy AI systems with autonomous decision-making capabilities that will fundamentally transform the way automation industries operate. Signaling a shift from traditional automation to intelligent agents capable of self-driven tasks. Businesses are rapidly embracing the power of AI, and at the forefront of this innovation we’ve got Agentic AI—a new frontier that brings us closer to truly autonomous decision-making systems.
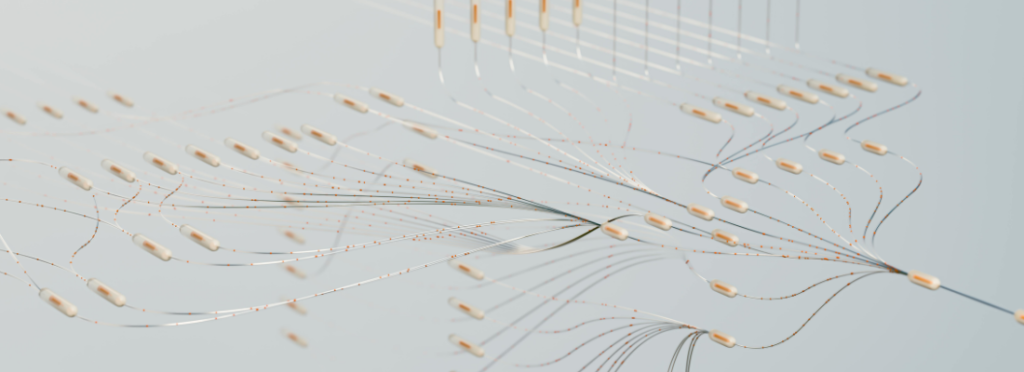
What is Agentic AI?
Agentic AI is a new technology that enables autonomous capabilities of a system. Agentic AI works on a principle of learning from experience and environment. It’s like training a robot to independently perform certain tasks with the need to explicitly say it. While this sounds like it’s taken out of a Sci-Fi movie, that’s exactly how Agentic AI works.
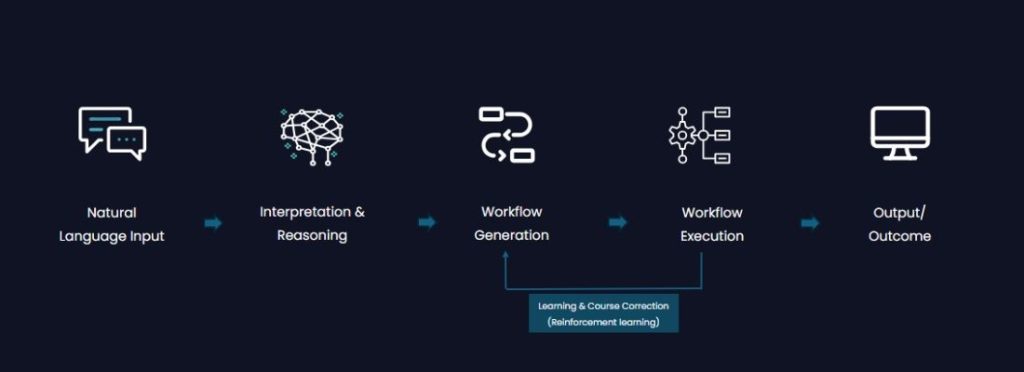
AI Agents are constantly learning from the data they receive and perform actions based on the context it is given. It also goes a step further to figure out the next steps as it performs a certain action, it learns how to do the tasks better each time. Agentic AI’s foundation lies in advanced machine learning (ML) algorithms, deep learning (DL), and reinforcement learning (RL), allowing it to continuously improve through interaction with data and environments.
Unlike its predecessors – AI Assistants, which were mostly reactive Agentic AI operates autonomously with a degree of agency—meaning it can make decisions, learn from its environment, and adjust its behavior without constant human oversight.
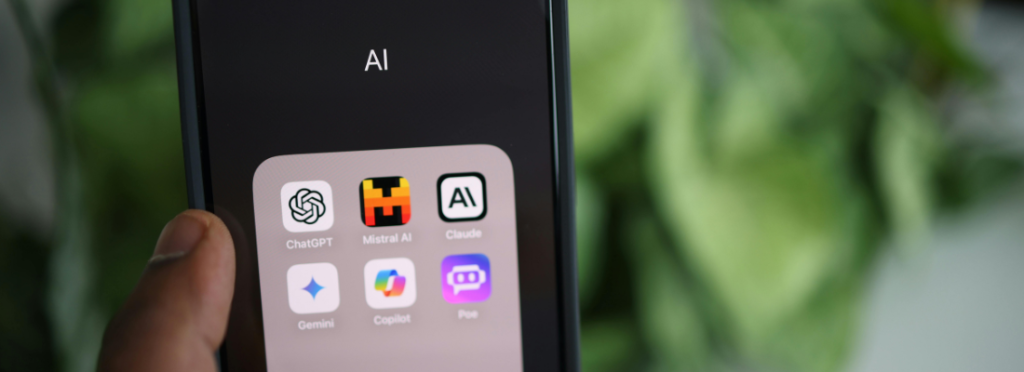
Evolution of AI – How Did We Get to Agentic AI?
If you have used a Tesla or seen one in action, you would think there’s nothing so fancy about it. But the decades of development that has gone into building the tech are just marvelous. While the task of driving may seem second nature to us humans, training machines to anticipate obstructions on the road, identify between road, gravel, trees and objects is a whole different ball game.
Tesla took almost 2 decades to develop this tech. Agentic AI has had a similar journey in some sense. If you look at it, autonomously driven cars thrive on the principles of Agentic AI. Agentic AI has evolved from simple, rule-based automation systems to sophisticated models capable of learning and decision-making. While in the early years of AI it was limited to constant human input. Today, this has changed over time. Thanks to advancements in machine learning (ML) and deep learning (DL), which allowed AI to learn from data and improve over time.
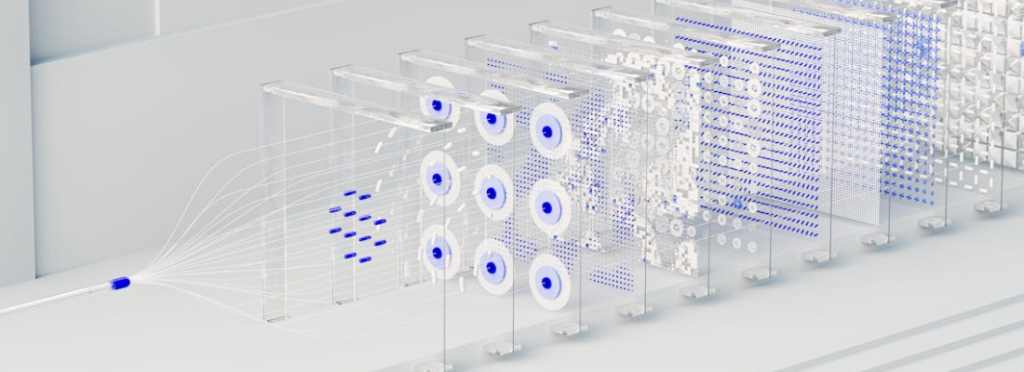
When we think of it. AI technology dates back to the 1990s and early 2000s where the rise of machine learning (ML) allowed AI to become more adaptive. With algorithms that could learn from data, systems began to perform tasks more efficiently. As deep learning matured, AI models like neural networks could mimic the brain’s cognition and unlock capabilities like image recognition, language translation, and autonomous driving.
Today, Large Language Models (LLMs) like OpenAI’s GPT and Google’s Bard are a shining example of this whole evolution. Combining the power of understanding, context, and decision-making into AI systems is what has led to display of higher degrees of autonomy in AI Agents. Reinforcement learning (RL) has enabled AI Agents to learn through trial and error, paving the way for Agentic AI—autonomous systems that make decisions and take action independently.
Differences Between Generative AI and Agentic AI
Generative AI, like ChatGPT and DALL-E, focuses on creating content. It is excellent at producing text, images, and even audio based on the patterns it has learned from massive datasets. However, it operates under human supervision. It’s a tool that assists but doesn’t take initiative on its own.
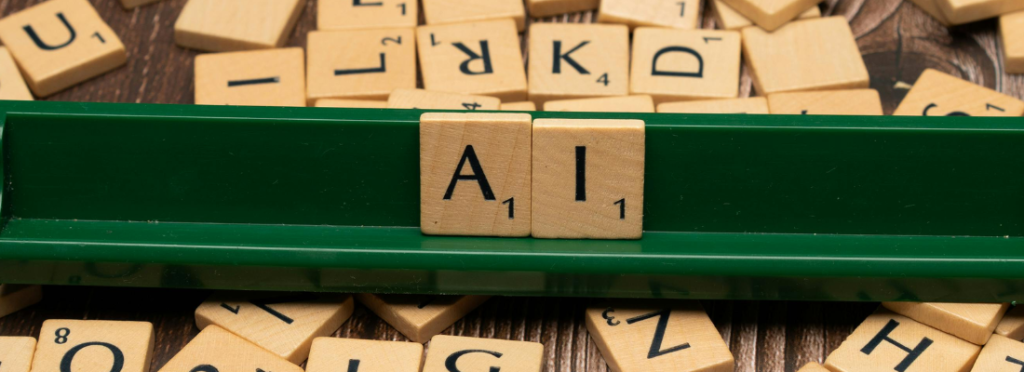
In contrast, Agentic AI extends beyond creation and assistance. It can initiate actions, make decisions, and drive processes without being prompted every step of the way. While generative AI acts as a creative aid, Agentic AI serves as an operational strategist—one that analyzes, predicts, and takes action based on the environment, much like a skilled professional would.
Aspect | Generative AI | Agentic AI |
Core Function | Creates content (text, images, audio) | Takes action and makes decisions autonomously |
Human Involvement | Requires prompts and supervision | Minimal to no supervision; acts independently |
Example | Tools like GPT, DALL-E | Autonomous app testing agents, self-driving systems |
Learning Capability | Learns from data to generate content based on patterns | Learns from data, environment, and adjusts actions to outcomes |
Output | Text, images, audio | Action-oriented results, decisions, process optimization |
Application | Content creation, customer service, data analysis | Autonomous testing, decision-making, process automation |
For businesses, this distinction is critical. Generative AI enhances efficiency in content and idea generation, but Agentic AI brings about true autonomy, transforming operational workflows.
How is Agentic AI Enabling Autonomous App Testing?
The implications of Agentic AI are especially groundbreaking in app testing. Traditionally, testing has been a resource-heavy process that requires human oversight at every stage—from scripting tests to analyzing results. Even with automation tools, a substantial amount of manual intervention is required to maintain, update, and optimize tests.
Agentic AI changes this by introducing autonomous app testing agents. These AI agents not only execute tests but also generate test cases based on user behavior, analyze performance metrics, and adjust testing strategies in real-time without the need for human involvement. Imagine a system where the AI is learning from each test run, understanding which areas of the app are prone to issues, and dynamically modifying the testing strategy accordingly.
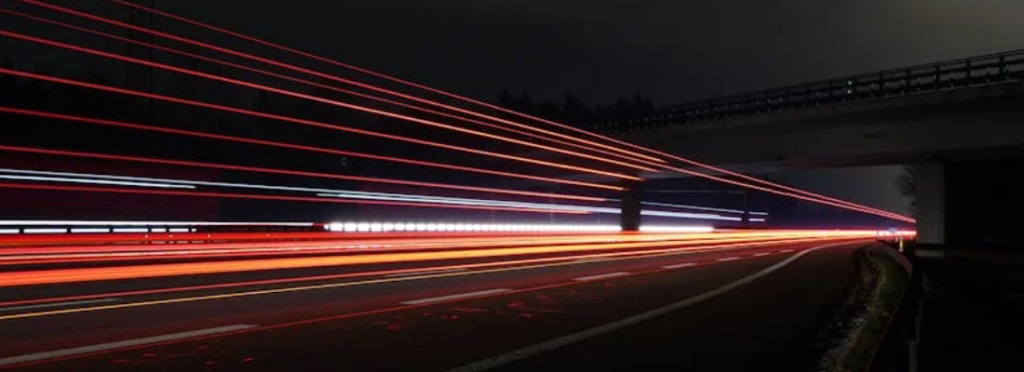
Deloitte highlights how this approach dramatically reduces testing time, improves accuracy, and allows businesses to launch apps faster while ensuring fewer defects in production. For businesses, this means lower costs, better user experiences, and a more agile development process.
Traditional Automation is Old Hat
For years, test automation has helped businesses streamline their testing processes. However, traditional automation is often rigid, requiring testers to create, manage, and update scripts for every feature change. According to Forrester, 56% of companies still struggle with outdated automation tools that can’t keep pace with continuous development cycles.
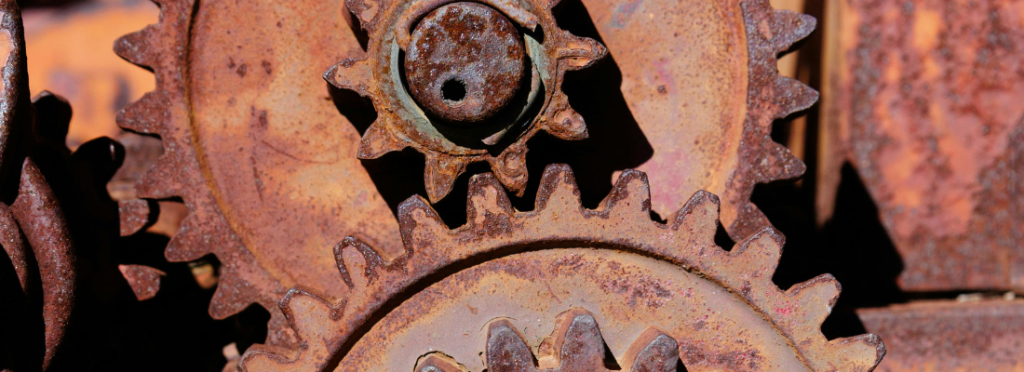
Traditional automation tools are task-focused and limited by the quality and quantity of input from humans. They are rule-based systems that require constant tweaking, prone to errors, and fail to adapt quickly in dynamic environments.
A New Solution: Autonomous Testing AI Agents
The next evolution in app testing is the integration of Agentic AI into testing workflows—resulting in Autonomous Testing AI Agents. These AI agents, powered by Agentic AI principles, can interpret natural language commands, write test scripts, execute them across multiple environments, and analyze results in real-time.
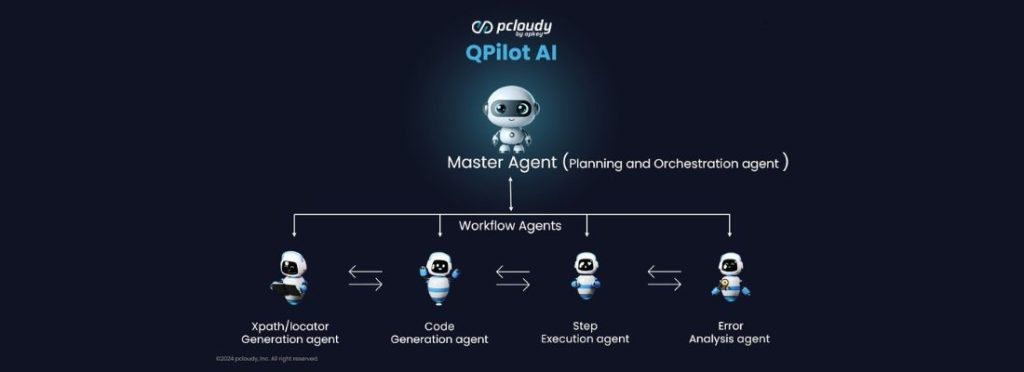
Pcloudy’s Qpilot.AI is a classic example of an App Testing AI Agent in action. It understands the testing requirements from the start, interprets, executes, verifies and validates every action that it performs, and finally gives us the results making it a truly autonomous AI Agent to perform your app testing. Unlike their traditional counterparts, autonomous testing agents learn from each test cycle, adjust based on new data, and continuously improve testing coverage.
Final Thoughts
It is still too early to predict how well AI Agents will perform in action. However, the self-learning aspect of AI Agents is what sets them apart and makes them indispensable in fast-paced development environments where apps are updated frequently, and new features are released constantly. Agentic AI doesn’t just automate; it optimizes. It doesn’t just run predefined tests; it creates new ones based on app usage patterns, minimizing human intervention and increasing the efficiency of the overall process.